Society & AI: Situational Power and Impact
From January to April 2019 I am involved in a seminar course on the Socio-Cultural and Political Implications of Artificial Intelligence (ARTS490). This article is part of a series of essays written for the seminar on AI’s implications for society.
A People’s Guide
Is machine learning having a disproportionate impact on people who are not involved in its creation?
In the Fall of 2018, Mimi Onuoha and Diane Nucera released A People’s Guide to AI to democratize access to information about artificial intelligence. The idea was to make it accessible to people from a wide range of backgrounds. Diane wanted to make sure that the 40% of people without internet in her hometown of Detroit could understand this new technology that would affect their lives.
We could consider those without internet as part of a larger group of people that lack situational power. They are unlikely to learn about machine learning through other means, so they won’t know how to measure and respond to its impact on them. Mimi shared that when it comes to the impact of artificial intelligence, those most affected are those who lack situational power.
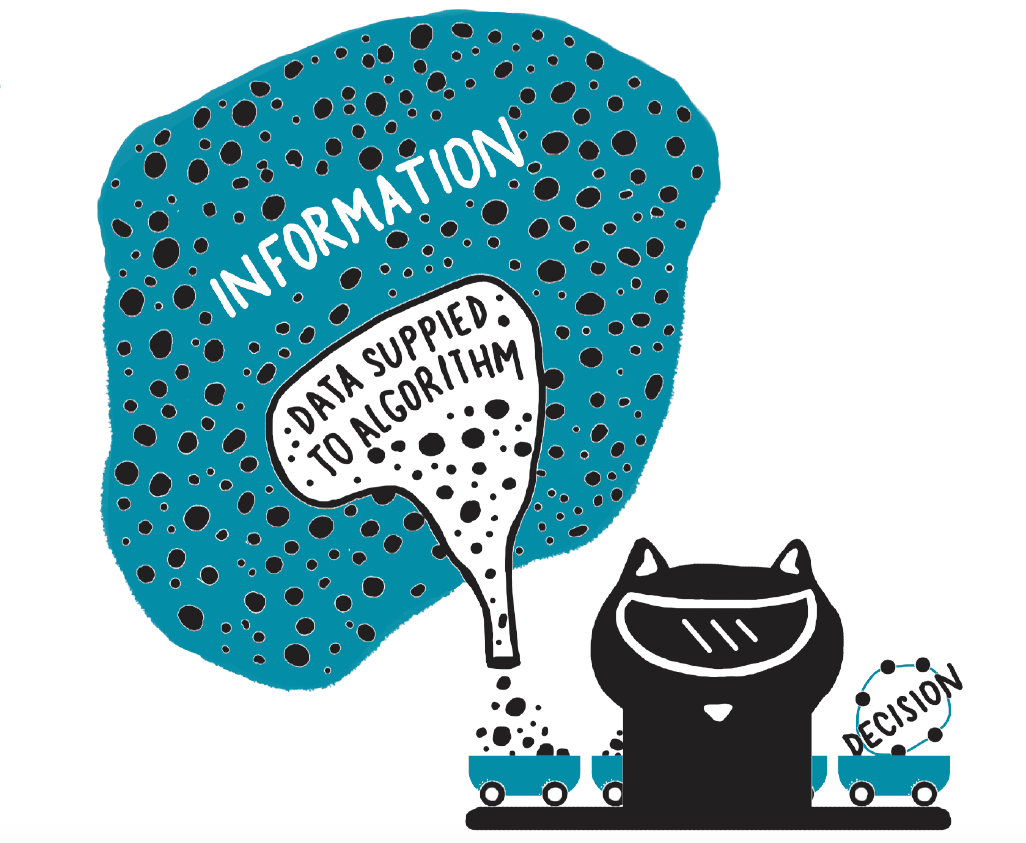
Situational Power
What is situational power? It is defined by Prabhakaran as the concept, “if there is another person such that he or she has power or authority over the first person in the context of a situation or task.” Situational power might diverge from the external power structure. How does this power manifest itself? It sometimes changes how two people talk to each other, the requests they make, and the expectations for response or action. For example, parents have legal authority over their children to make decisions for them, but in some situations the children have some power over their parents (e.g. when crying in a restaurant).
There are two relationships where situational power matters when it comes to AI. The first is in a group’s interactions with other human groups. For example, a jury has situational power over someone being charged with a crime. The second is in the relationship between humans and the machine learning models themselves.
Having situational power in a relationship with a machine learning model would mean having the ability to understand and manipulate the model. Many people who are affected by models used in areas like criminal justice or public policy lack situational power. Even the ones using the output of the model can find themselves in a power imbalance that can negatively affect their work.
Those most affected are those who lack situational power - Mimi Onuoha
When you first read the People’s Guide to AI you may be tempted to think it is only meant for marginalized people who have little access to technology and little background in the field of computer science. However, the situational power framework shows us that the field of people affected by a lack of understanding of AI is actually much broader. Take the examples of judges and police officers, jobs that typically are associated with a large amount of power. Often these individuals lack situational power in their relationships with the software they use.
David Robinson analyzed the understanding of machine learning models in his 2018 journal paper The Challenges of Prediction: Lessons from Criminal Justice. He interviews a police officer who says about the Beware software system tested in Fresno in 2015, “We don’t know what their algorithm is exactly… We don’t have any kind of a list that will tell us, this is their criteria.” In the Wisconsin Supreme Court, he finds that judges didn’t understand the pre-trial analysis software they were using in sentencing decisions.
It is clear that there is a power imbalance between the software we use and the people using it and being affected by its outcomes. As developers of machine learning it is our responsibility to both decrease this power imbalance, as well as protect those with less power from being exploited.
Impact
Unfortunately, it is already clear that the ways machine learning is being applied will not just affect people, but will do so unjustly. Safiya Umoja Noble’s book Algorithms of Oppression catalogues the multitude of negative impacts of search engines on black women. She finds that many minority groups and people who have been historically oppressed have that oppression reinforced by organizations whose algorithms don’t consider the social impact of their output.
Noble cites an example from Google Image search to highlight this where searching for ‘unprofessional hairstyles’ turns up photos of black women while ‘professional hairstyles’ returns mostly photos of white women.
A quick example (you can find the original here) cited in the book:
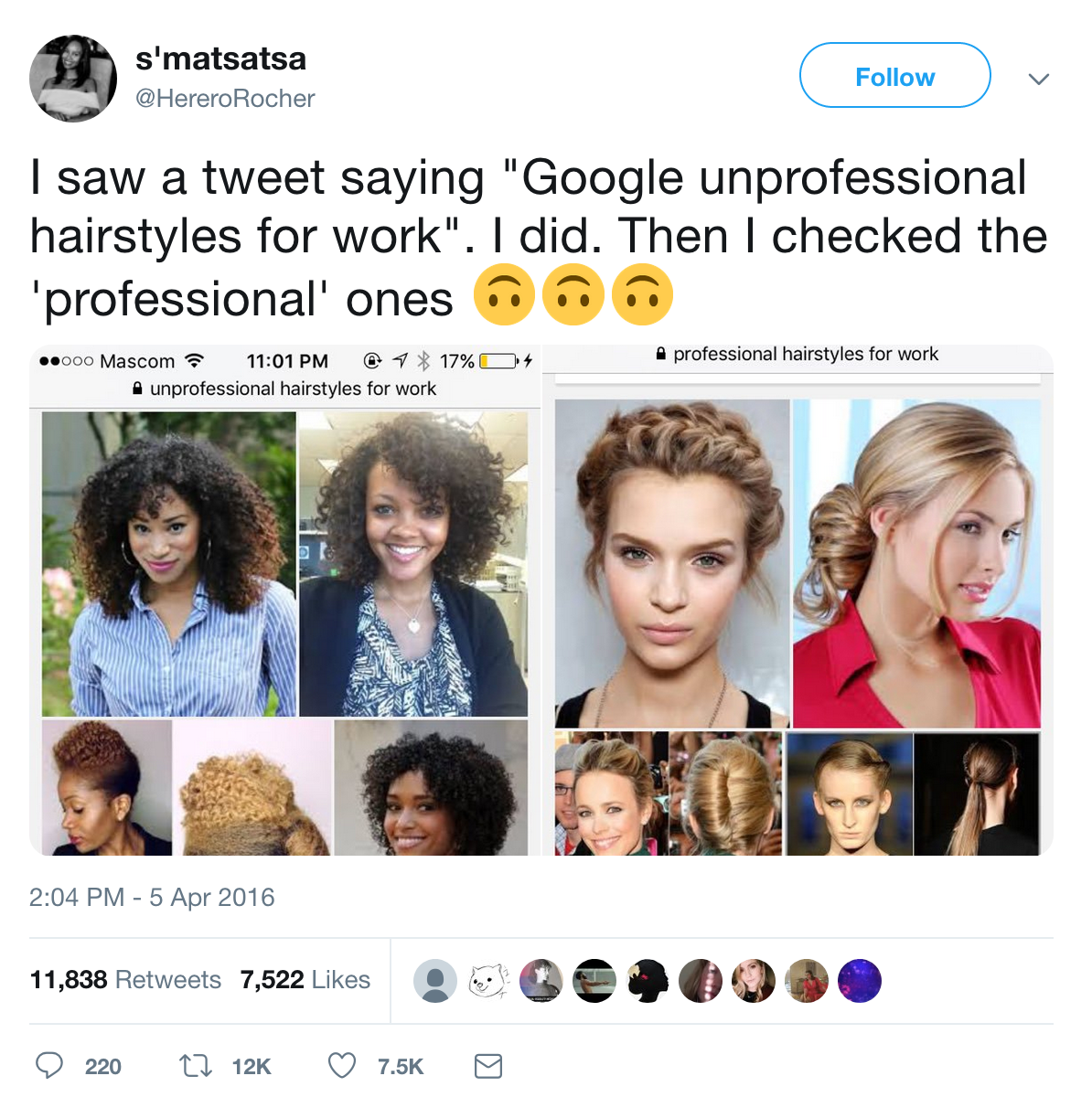
These are obvious problems that we need to solve, not just for search, but for all applications of AI. I find myself drawn to both the work researchers like Mimi and Safiya have done to highlight these issues. But I wonder how we can codify ways of protecting groups that lack situational power from experiencing injustice.
Measuring Impact with a Bias Framework
I believe the recent work of Harini Suresh and John Guttag to catalogue different kinds of bias will help. They consider five different kinds of bias that are introduced to machine learning models at different points in the data/training pipeline. The five biases are:
- Historical Bias: from how the data is generated
- Representation Bias: from what portion of the general population is found in the chosen dataset
- Measurement Bias: from the choices made of what to measure about the population
- Evaluation Bias: from how the benchmarks chosen to improve model performance skew the output
- Aggregation Bias: from how the model is applied or used
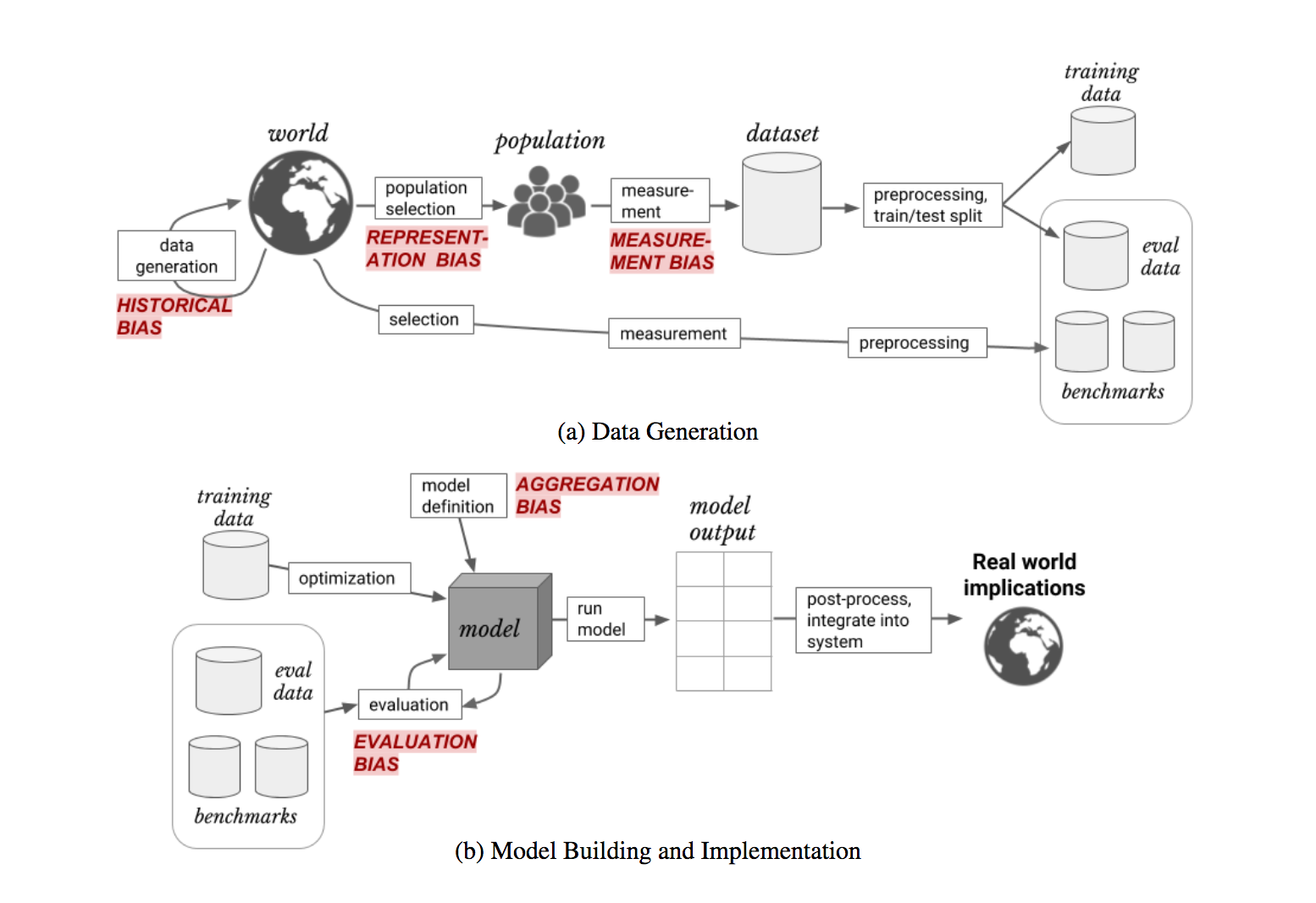
A good extension of this work would be to measure the links between each kind of bias and the outcomes that Safiya Noble highlights. In general she seems to talk about historical, representation, and aggregation bias, but it could be interesting to examine all five in more detail.
We should continue to acknowledge the power imbalances that come with the application of machine learning while we actively learn to maintain justice in how the models affect people.